Neural network theory evolution as an innovative factor of successful and dynamic development of economic systems
neural networks basing on the study of the main neural network application trends in economics, in particular, the application of artificial neural networks in economics of foreign countries. Analyzing the specifics of the neural network application in economics, and also studying their strengths and weaknesses, the authors concluded that the Russian market of neural network solutions was poorly developed due to insufficient research funding. The conclusion follows from the above mentioned that at present the application of neural networks in various spheres of economics is an actual topic for research.
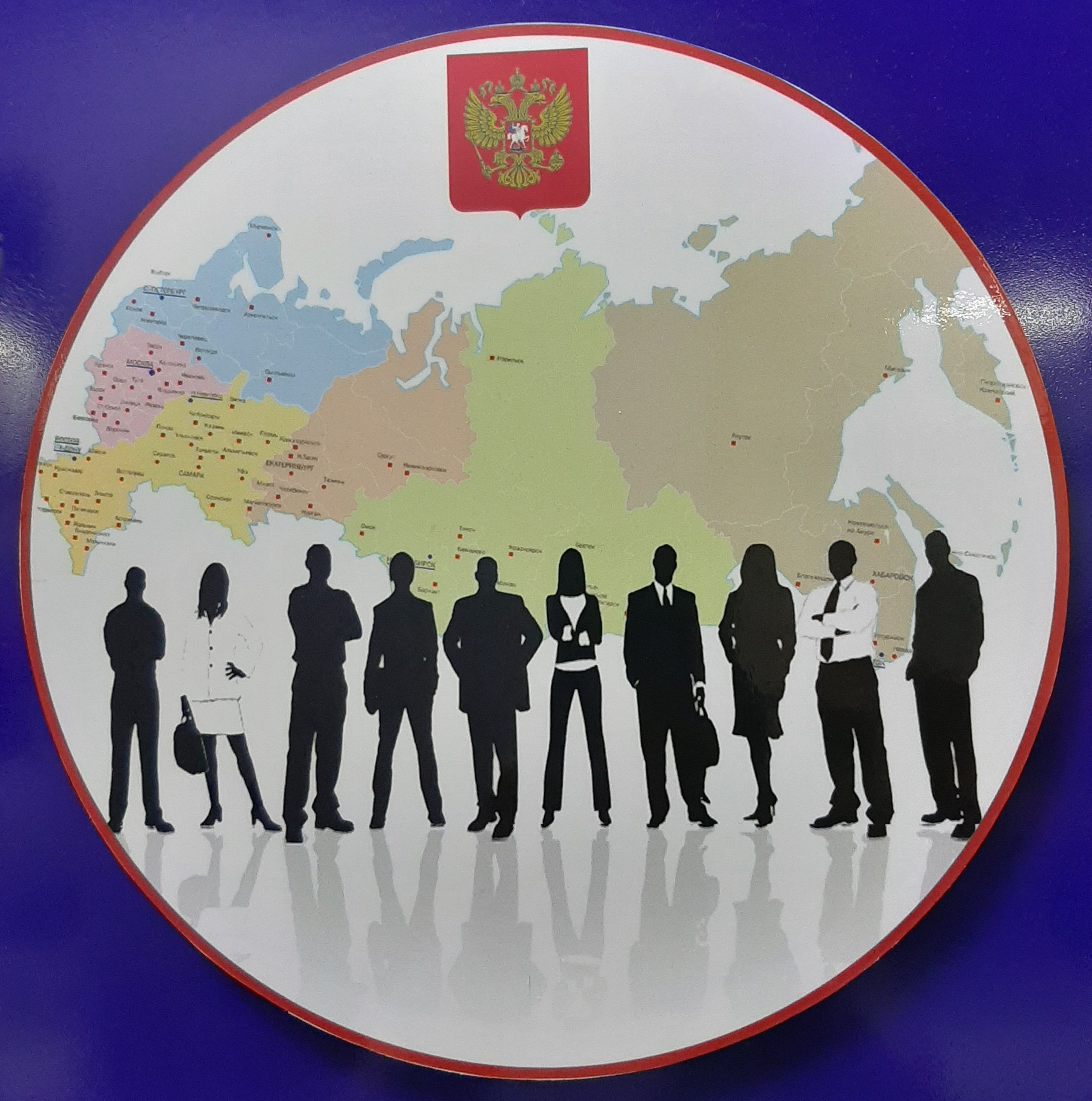
In the management process, the application of neural networks became possible at the beginning of the 20th century; it is based on the properties and regularities of functioning of nerve cells – neurons (Hopfield 1982; McCulloch & Pitts 1943; Hebb 1949). Due to neurons, the entire system of vital activity control is realized; more specifically, such control is performed through their interaction and numerous connections forming a branched network through which electrochemical signals propagate (Rosenblatt 1962; Anderson 1972; Grossberg 1976).
Camillo Golgi and Santiago Ramon y Cajal were the founders of the ongoing research. In 1906, they discovered neurons to the scientific world (Babmindra & Vorobyov 1985). However, initially neural networks were studied only in the field of biology and physics (Widrow & Hoff 1961; Hecht-Nielsen 1987; Kohonen 1972; Kohonen 1982).
The next stage in the development of this science was the invention of artificial neural networks, represented in the form of mathematical models and implemented in the form of software products and devices. These models were based on the biological neuron constructing principle. The first developments in the field of production and application of artificial neural networks were carried out by the scientists Warren Sturgis McCulloch and Walter Pitts in 1943 (McCulloch & Pitts 1956). They could provide solutions to issues with no dependence between input and output data, solution algorithms, which made it impossible to use traditional mathematical calculations or expert systems.
The first artificial neural network – perceptron – was invented by Frank Rosenblatt in 1958 to model the activity of the human brain when processing visual data and in learning to recognize objects (Rosenblatt 1958; Rosenblatt 1960; Joseph 1960).
At the end of the 20th century, neural networks began to be used in the field of forecasting economic processes. Today, various approaches to the application of neural networks in the management of economic subjects are being studied by scientists such as Tokarev, K.E. (2014, 2016), Guba, K.A., & Dubanov, G.N. (2014), Sokolov, A.F. (2011), Rogachev, A.F., & Kuzmin, V.A. (2013), Rogachev, A.F., & Shubnov, M.G. (2012), Rogachev, A.F., & Shubnov, M.G. (2013), Shatyrko, D.V., Tokarev, K.E., & Kuzmin, V.A. (2015), Astafieva, N.M. (2004), Galushkin, A.I. (2010), Dorogov, A.Yu. (2006), Redko, V.G. (2011), Terekhov, V.A., Efimov, D.V., & Tyukin, I.Yu. (2002), Chervyakov, N.I., Sakhnyuk, P.A., Shaposhnikov, A.V., & Makokha, A.N. (2003), Beer, C. (1993). The founder of the application of neural networks in the field of economic management is the English cyberneticist Stafford Beer, who proposed principles of management based on neurophysiological mechanisms (Vissema 2000). An artificial neural network is a computer system simulating the activity of biological neurons with parallel information processing, the ability to learn and summarize the accumulated knowledge (Tokarev 2016).
The idea of creating and modeling the principles of self-organization of economic systems was developed by A.I. Ivakhnenko, who developed a model for the development of the economy of England, as well as for assessing and forecasting the state of the economies of countries such as the USA, Bulgaria, and Germany (Ivakhnenko & Muller 1985). Shchetinin V.G. also worked in this field; he built a neural network for modeling and solving problems in analyzing the economics of Mordovia and the Penza Region (Schetinin & Kostyunin 1998).
Nevertheless, in the management of economic entities, there is no uniform methodology for the formation of the architecture and parameters of neural networks, which would take into account their specifics. This is due to the specifics of the economic management system, its instability and a wide range of interactions, as well as the range of applications.
Special software packages are installed in state institutions and commercial enterprises, holdings, credit institutions, financial and insurance companies. Currently, they are used to forecast macroeconomic indicators; forecast calculations of an enterprise's activity; in financial transactions, including exchange and market indices, exchange rates; for analytical studies to make business decisions; valuation of enterprises; for estimating enterprises' work quality.
The application of artificial neural networks in economics has successfully proved itself abroad. Today on the basis of artificial neural networks, the programs have been designed for price forecasts of exchange rates, securities portfolio analysis, securities trading, which are used by stock exchanges in Japan, the United States, and the United Kingdom (Safonenkov 2015). The United Nations apply self-organizing maps (the Kohonen maps) to rank countries by the poverty level (Ostroukhov & Panfilov, n.d.). The positive results of artificial neural networks served as an incentive for the joint work of the London Business School, the University College London, TSB Bank Technology and the Henley Centre for Forecasting for the creation and integration of the Neuroforecasting Club and Neural Networks for Financial Services programs. The services of these programs are used by large financial corporations in Great Britain, France, Germany, Japan, the USA, Canada, and Australia (Tsuprikov 1985), since they are covered by a database and modeled by a network of neurons separately for each geographic location, at the initial stage for further integration in the Control Centre. The well-known financial corporation Citicorp uses them for analysis and short-term forecasting of currency fluctuations (Kosharova 2013).
The application of neural networks for the analysis of the real estate market allowed the Richard Borst company to increase the turnover of capital in some branches by 6% (ToraCenter, n.d.). Microsoft uses neural networks to construct the ratings of their customers, and to create a distribution base for advertising mailings, which reduced advertising costs by 15%. Artificial intelligence is also used to predict the bankruptcy by worldwide large banks, such well-known corporations as General Electric, American Airlines, Coca Cola, Philip Morris, Procter & Gamble, Merrill Lynch, Ford, Reno and many others.
Figure 1 Dynamics of the distribution of patents in the field of neural network development and application among countries for 2004-2014 (Public Analytical Report on the Direction of Neurotechnology, 2014)
The Russian market of neural network developments is only evolving, despite the negative trend that occurred during the last decade (Figure 1).
Among other causes, the current dynamics can be explained by the lack of targeted public funding for scientific research in the field of neural networks.
Materials and methods. When writing this paper, general scientific methods were used, such as empirical research, theoretical cognition, general logic methods and techniques; as well as methods of system analysis, the method of comparisons and analogies, the method of generalizations, etc.
The authors used the research results outlined in the works of leading Russian and foreign scientists studying neural networks in various branches of science.
The research methodology was based on up-to-date methods of information collection, processing and analysis.
- Results
As a result of the analysis, the authors came to the conclusion that the creation of artificial neural networks made it possible to carry out the assigned tasks, the solution of which had not previously been possible; these tasks could be grouped as follows:
1 Modeling for an object of one of its target attributes;
2 Modeling of the value of a numerical attribute for an object;
3 Dynamic forecasting of the value of a numerical attribute of an object, using time measurements of the values of the same attribute;
4 Automatic grouping of objects.
The authors' management system development approach proved its effectiveness when used in large production associations, as well as the economy of the country as a whole. The authors have grouped the main trends in the application of neural networks in economics, which is presented in Table 1.
Table 1 The main trends in application of neural networks in economics (Compiled by the authors)
The analysis of the data on the development of investment foreign programs shows that governments all over the world pay great attention to projects for the neural technologies research (Table 2), which can be adapted and applied in the economics.
Table 2 The largest foreign government investment programs for financing global neural network research projects (Compiled from the materials (Public Analytical Report on the Direction of Neurotechnology, 2014)
Scientific developments in the field of neural technologies require significant (million) amounts of investments, as this type of research is based on the purchase and creation of up-to-date processors and technological equipment. The data presented in Table 2 explain the trend in the development and application of neural networks (Figure 1). As we see, the investment leaders in the world market are the United States and the European Union. The policy of the Chinese government regarding the relevance and effectiveness of investments in the development of neural networks has been formed over the past three years.
Russian enterprises, including large holdings, cannot compete with foreign governments at the level of the investment activity; therefore, the government financing is required. To date, a number of developments, processors for the creation of neural network software are purchased abroad by Russian enterprises. The joint financing of the neural technology scientific research is possible. In the event of the joint financing, the government projects will be implemented to ensure the development of medical technologies, improve information technologies in the field of treasury, budget and tax systems. The research will be conducted through private financing in order to develop software to solve economic problems of a commercial nature.
At present, the following Russian universities are engaged in the creation of new neural software products, including in the field of solving economic issues: Moscow Institute of Physics and Technology (MIPT), Lomonosov Moscow State University (MSU), National Research University Higher School of Economics (HSE), Skolkovo Institute of Science and Technology (Skoltech), etc., as well as such large companies as Mail.Ru Group Holding, Yandex; Tora-Center has a special importance in this list, which has developed software products for more than 700 companies and credit organizations.
Today in Russia, neural networks are used in more than 30 credit organizations and 60 financial companies. At the same time, they are also used to increase the efficiency of government public services, such as the Ministry of Emergency Situations, tax authorities, and the Central Bank of Russia (Tora-Center, n.d.).
The conducted analysis made it possible to identify the main trends and features of the application of neural networks in economics of foreign countries (Table 3).
Table 3 Features of artificial neural network application in economics of foreign countries (Compiled by the authors)
- Discussion
The following main advantages of neural networks can be identified explaining the increase in the activity of their use in economics:
1 Easy to use and operate;
2 Compatibility with a variety of software and databases;
3 Ability to self-learning and self-setting when editing individual parameters;
4 Ability to perform their functions in the absence of some data, the presence of distorted and inconsistent information;
5 Accounting for the impact of external factors (interference) on the cluster data system (Kokh & Prosalova 2017);
6 Ability to operate with a large number of variables while not reducing the accuracy of calculations when they increase;
7 An increase in the speed of data processing by increasing the volume of internal information.
In addition to these advantages, neural networks have a number of disadvantages:
1 Lack of standard calculation mechanisms for specific tasks. When setting up a new specific task, it is necessary to independently develop a calculation model for the neural network and form the basis for its learning.
2 The formalization complexity of processes, the neural network. The processes occurring during the operation of the neural network are quite complex for understanding, both for ordinary users and for developers, so it often remains the so-called "black box".
3 The formation complexity of an information base for learning. Before the neural network operates, it needs to be trained on specific patterns. The instability conditions typical for the economic system do not allow accumulating a sufficiently large database over a long period of time.
Nevertheless, it is necessary to highlight the unique property of neural networks, their ability to take into account and model the change in economic processes, as well as the behavior of society, something that cannot be calculated using mathematical models.
This property of neural networks determines the effectiveness of their use in predicting the activity of a cluster. The cluster management is a process of organizing, controlling and stimulating the system activity that is influenced both by internal and external factors. The fact that the cluster consists of a large number of participants, which differ both in the field of activity and in their organizational and legal form, exacerbates the special impact. As a result, the probability of losing control and disorganizing the centralized management system increases.
- Conclusion
Based on the above research, the authors proposed the definition of an artificial neural network applied in economic activity as a computer system that simulated changes in economic processes, as well as the behavior of society, and had the ability to learn and summarize the accumulated knowledge.
It should be noted that the application of neural networks in all areas of human activity is moving at an increasing pace. At the initial stage, the neural networks were considered only in such narrow spheres as physics and biology; while at the present stage, the neural networks introduction occurs practically in all scientific fields. This is due to the fact that the neural network application makes it possible to analyze the input information, and also to find patterns in it, to make predictions. Thus, the neural networks represent a new and very promising computational technology, which gives completely new approaches to the study of various issues in the economic field.
Acknowledgements
The authors are grateful to Viktor Nikolaevich Likhovidov, Associate Professor of the Department of Applied Mathematics, Mechanics, Management and Software at the Far Eastern Federal University, and Yury Vladimirovich Rozhkov, Ph.D. in Economics, Professor of the Khabarovsk State University of Economics and Law, for valuable advice in planning the present research and recommendations for the design of the paper.
References
Anderson, J.A. (1972). A Simple Neural Network Generating an Interactive Memory. Mathematical Biosciences, 14, 197–220.
Astafieva, N.M. (2004). Veivlet analiz: osnovy teorii i primery primeneniya [Wavelet Analysis: The Theory Fundamentals and Examples of Applications]. Uspekhi fizicheskikh nauk, 166, 1145-1170.
Babmindra, V.P., & Vorobyov, V.S. (1985). Santyago Ramon-i-Kakhal i ego rol v sozdanii sovremennoi neirobiologii (k 50-letiyu so dnya smerti) [Santiago Ramon y Cajal and His Role in the Creation of Modern Neuroscience (to the 50th Anniversary of His Death)]. Arkhiv anatomii, gistologii i embriologii, 88(2), 87‑92.
Beer, C. (1993). Mozg firmy [Brain of the Firm]. Moscow: Radio i svyaz. (p. 524).
Chervyakov, N.I., Sakhnyuk, P.A., Shaposhnikov, A.V., & Makokha, A.N. (2003). Neirokompyutery v ostatochnykh klassakh [Neurocomputers in the Residual Classes] (Book 11). Moscow: Radiotekhnika. (p. 272).
Dorogov, A.Yu. (2006). Sistemnye invarianty bystrykh perestraivaemykh preobrazovanii [System Invariants of Fast Tunable Transformations]. In Sovremennye problemy neiroinformatiki [Modern Issues of Neuroinformatics] (Book 23, Part 1, pp. 43-50). Moscow: Radiotekhnika.
Galushkin, A.I. (2010). Neironnye seti: osnovy teorii [Neural Networks: The Theory Fundamentals]. Moscow: Goryachaya liniya-Telekom. (p. 496). Grossberg, S. (1976).
Adaptive Pattern Classification and Universal Recoding: I. Parallel Development and Coding of Neural Feature Detectors. Biological Cybernetics, 23, 121-134.
Guba, K.A., & Dubanov, G.N. (2014). Povyshenie effektivnosti sistemy prinyatiya upravlencheskikh reshenii za schet primeneniya apparata neironnykh setei [Increase of the Managerial Decision System Effectiveness due to Neural Network Application]. Biznes. Obrazovanie. Pravo. Vestnik Volgogradskogo instituta biznesa, 3(28), 84-87.
Hebb, D.O. (1949). The Organization of Behavior: A Neuropsychological Theory. New York: Psychology Press.
Hecht-Nielsen, R. (1987). Kolmogorovs Mapping Neural Network Existence Theorem. In IEEE First Annual International Conference on Neural Networks (Vol. 3, pp. 11-13). San Diego. Hopfield, J.J. (1982). Neural Networks and Physical Systems with Emergent Collective Computational Abilities. Proceedings of National Academy of Sciences, 79, 2554-2558.
Ivakhnenko, A.G., & Muller, J.A. (1985). Samoorganizatsiya prognoziruyushchikh modelei [Self-Organization of Predictive Models]. Kiev: Tekhnika. (p. 225).
Joseph, R.G. (1960). On Predicting Perceptron Performance. Investigative Reporters and Editors Conference, 2, 71-72.
Kohonen, T. (1972). Correlation Matrix Memories. IEEE Transactions on Computers, C-21(4), 353-359.
Kohonen, T. (1982). Self-Organized Formation of topologically Correct Feature Maps. Biological Cybernetics, 43, 59–69.
Kokh, L.V., & Prosalova, V.S. (2017). Innovatsionnaya deyatelnost klasterov v Rossii i printsipy ee osushchestvleniya [Innovative Activity of Clusters in Russia and the Principles of Its Implementation]. Vestnik Zabaikalskogo gosudarstvennogo universiteta, 23(4), 102-109.
Kosharova, N.B. (2013). Ponyatie iskusstvennykh neironnykh setei i preimushchestvo ikh ispolzovaniya v reshenii ekonomicheskikh zadach [The Concept of Artificial Neural Networks and the Advantage of Their Use in Solving Economic Problems]. Ekonomika i predprinimatelstvo, 12 (Part 3), 430-432.
McCulloch, W.S, & Pitts, W. (1956). Logicheskoe ischislenie idei, otnosyashchikhsya k nervnoi aktivnosti [A Logical Calculus of the Ideas Immanent in Nervous Activity]. In C.E. Shannon, & J. McCarthy (Eds.), Avtomaty [Automata Studies] (Trans. from English, pp. 363- 384). Moscow: Izdatelstvo inostrannoi literatury.
McCulloch, W.S., & Pitts, W. (1943). A Logical Calculus of the Ideas Immanent in Nervous Activity. The Bulletin of Mathematical Biophysics, 5(4), 115-133.
Ostroukhov, I., & Panfilov, P. (n.d.). Neiroseti: karty Kokhonena [Neural Networks: Kohonen Maps]. Retrieved February 27, 2017, from http://www.tora-centre.ru/papers.htm
Publichnyi analiticheskii doklad po napravleniyu "Neirotekhnologii" [Public Analytical Report on the Direction of Neurotechnology]. (2014). Retrieved February 27, 2017, from [Electronic source] Access mode: https://reestr.extech.ru/docs/analytic/reports/neuroscience.pdf
Redko, V.G. (2011). Evolyutsiya, neironnye seti, intellekt: Modeli i kontseptsii evolyutsionnoi kibernetiki [Evolution, Neural Networks, Intellect: Models and Concepts of Evolutionary Cybernetics]. Moscow: LIBROKOM. (p. 224).
Rogachev, A.F., & Kuzmin, V.A. (2013). Modelirovanie ekologo-ekonomicheskikh sistem s ispolzovaniem algoritmov nechetkogo vyvoda [Modeling of Ecological and Economic Systems Using Fuzzy Inference Algorithms]. Izvestiya Nizhnevolzhskogo agrouniversitetskogo kompleksa: Nauka i vysshee professionalnoe obrazovanie, 1, 230-235.
Rogachev, A.F., & Shubnov, M.G. (2012). Otsenka prognoznogo urovnya urozhainosti na osnove neirosetevykh modelei dinamiki [Estimation of the Forecasted Yield Level on the Basis of Neural Network Dynamics Models]. Izvestiya Nizhnevolzhskogo agrouniversitetskogo kompleksa: Nauka i vysshee professional'noe obrazovanie, 4, 226-231.
Rogachev, A.F., & Shubnov, M.G. (2013). Postroenie neirosetevykh modelei prognozirovaniya vremennykh ryadov urozhainosti na osnove avtokorrelyatsionnykh funktsii [Construction of Neural Network Models of Forecasting Time Series of Productivity Based on Autocorrelation Functions]. Sovremennye problemy nauki i obrazovaniya, 5. Retrieved January 23, 2016, from www.science-education.ru/111-10688.
Rosenblatt, F. (1958). The Perceptron: A Probabilistic Model for Information Storage and Organization in the Brain. Psychological Review, 65, 386‑408.
Rosenblatt, F. (1960). Perceptron Simulation Experiments. Investigative Reporters and Editors Conference, 48(3), 301-309.
Rosenblatt, F. (1962). Principles of Neurodynamics. New York: Spartan.
Safonenkov, A.A. (2015). Primenenie iskustvennykh neironnykh setei v finansovoi sfere [Application of Artificial Neural Networks in the Financial Sphere]. In Nauchnoe soobshchestvo studentov XXI stoletiya: Ekonomicheskie nauki: sb. st. po mat. XXXIII mezhdunar. stud. nauch.-prakt. konf. [Scientific community of students of the 21st century. Economic Sciences: Collection of Articles Based on Proceedings of the XXXIII International Students' Scientific-Practical Conference No. 6(33)]. Novosibirsk. Retrieved February 13, 2017, from http://sibac.info/archive/economics/6(33).pdf
Schetinin, V.G., & Kostyunin, A.V. (1998). Prinyatie reshenii na neironnykh setyakh optimalnoi slozhnosti [Decision Making on Neural Networks of Optimal Complexity]. Avtomatizatsiya i sovremennye tekhnologii, 4, 38-43.
Shatyrko, D.V., Tokarev, K.E., & Kuzmin, V.A. (2015). Modelirovanie ekonomicheskogo razvitiya regionalnogo APK s ispolzovaniem instrumentalnykh sred nechetkogo logicheskogo vyvoda [Modeling of Economic Development of the Regional Agro-Industrial Complex Using Tool Environments of Fuzzy Inference]. Fundamentalnye issledovaniya, 7(1), 217-221.
Sokolov, A.F. (2011). Ekonometricheskaya model vliyaniya sotsialno-ekonomicheskikh faktorov na effektivnost regionalnogo upravleniya [Econometric Model of the Influence of Socio-Economic Factors on the Effectiveness of Regional Management]. Biznes. Obrazovanie. Pravo. Vestnik Volgogradskogo instituta biznesa, 4(17), 79-82.
Terekhov, V.A., Efimov, D.V., & Tyukin, I.Yu. (2002). Neirosetevye sistemy upravleniya. Kn. 8: Ucheb. posobie dlya vuzov [Neural Network Management Systems. Book 8: Textbook for Universities]. Moscow: IPRZHR. (p. 480).
Tokarev, K.E. (2014). Primenenie nechetkikh neirosetevykh tekhnologii v zadachakh modelirovaniya diagnosticheskoi deyatelnosti vracha [Application of Fuzzy Neural Network Technologies in the Tasks of Modeling the Diagnostic Activity of a Physician]. In Nauchnye osnovy strategii razvitiya APK i selskikh territorii v usloviyakh VTO. Materialy Mezhdunarodnoi nauchno-prakticheskoi konferentsii [Scientific Fundamentals of the Strategy for the Development of the Agro-Industrial Complex and Rural Territories in the Context of the WTO. Proceedings of the International Scientific and Practical Conference] (pp. 197- 200). Volgograd: VolSAU.
Tokarev, K.E. (2016). Neirosetevye metody i algoritmy upravleniya v sotsialnoekonomicheskikh sistemakh [Neural Network Methods and Control Algorithms in SocioEconomic Systems]. Biznes. Obrazovanie. Pravo. Vestnik Volgogradskogo instituta biznesa, 2(35), 124-127.
Tsuprikov, S. (1985). Neironnye vychisleniya berutsya na vooruzhenie finansistami [Neural Computing is Used for Finance]. Computerworld Moscow, 7, 58-59.
Vissema, H. (2000). Strategicheskii menedzhment i predprinimatelstvo: vozmozhnosti dlya budushchego protsvetaniya [Strategic Management and Entrepreneurship: Possibilities of Future Prosperity] (Trans. from English). Moscow: Finpress. (p. 272).
Widrow, B., & Hoff, M.E. (1961). Adaptive Switching Circuits. In 1960 IRE WESCON Convention Record (pp. 96-104). IRE. Tora-Tsentr [Tora-Center]. (n.d.). Retrieved October 20, 2016, from http://www.toracentre.ru
- Federal State Budgetary Educational Institution of Higher Education "Saint-Petersburg State Marine Technical University", 190121, Russia, St. Petersburg, Lotsmanskaya Str., 3
- The Branch of Federal State Budgetary Educational Institution of Higher Education "Vladivostok State University of Economics and Service" in Nakhodka, 692902, Russia, Nakhodka, Ozernaya Str., 2
- Federal State Budgetary Educational Institution of Higher Education "Vladivostok State University of Economics and Service", 690014, Russia, Vladivostok, Gogolya Str., 41
- Federal State Budgetary Educational Institution of Higher Education "Vladivostok State University of Economics and Service", 690014, Russia, Vladivostok, Gogolya Str., 41
- Federal State Budgetary Educational Institution of Higher Education "Vladivostok State University of Economics and Service", 690014, Russia, Vladivostok, Gogolya Str., 41, E-mail: asya-nikitina@mail.ru